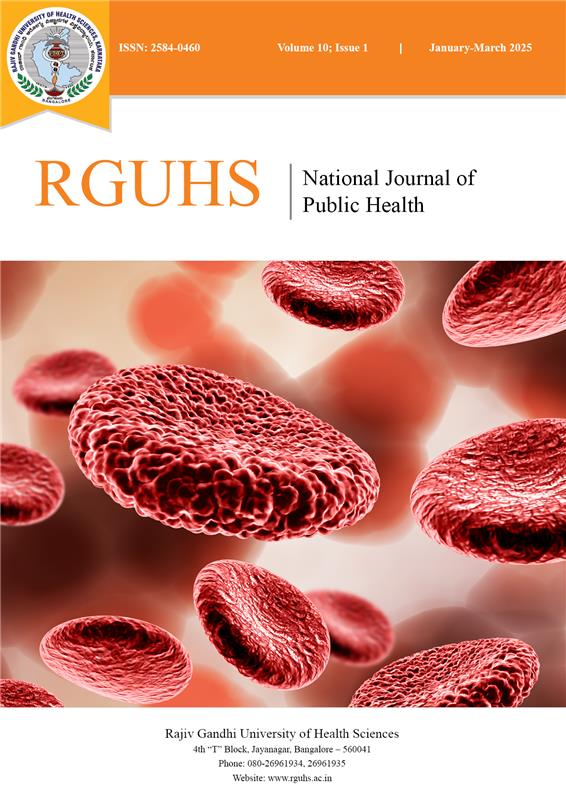
RGUHS Nat. J. Pub. Heal. Sci Vol No: 10 Issue No: 1 eISSN: 2584-0460
Dear Authors,
We invite you to watch this comprehensive video guide on the process of submitting your article online. This video will provide you with step-by-step instructions to ensure a smooth and successful submission.
Thank you for your attention and cooperation.
NS Murthy,1 C. Chandrashekara Nooyi2 , K Radhika3 , N S Shivaraj4
1: Research Director, DRP and Professor and Research Coordinator, M.S. Ramaiah Medical College & Hospitals (MSRMC),MSR Nagar, MSRIT Post, Bangalore-560054. (Ex. Emeritus Medical Scientist, ICMR, New Delhi, Deputy Director ( Sr. Grade), 2: Professor and Head, Department of Community Medicine, 3: Statistician cum Lecturer, 4: Assistant Professor, (Bio-Statistics), Department of Community Medicine, MS Ramaiah Medical College, Bangalore,
Address for correspondence:
C.Chandrashekara Nooyi
Professor and Head, Department of Community Medicine, MSRMC, Bangalore.
Email: chandrashekara shalinicnooyi@gmail.com

Abstract
None
Keywords
Downloads
-
1FullTextPDF
Article
Introduction
Bio-statistics has played an important role in the development of medical and biological sciences as well as in the development of various disease control and prevention measures. The discipline of biostatistics is nowadays a fundamental scientific component of biomedical, public health and health services research. Over the last few decades, bio-statistics have become more quantitative, stochastic, evidenced based with the growth of medical sciences and public health oriented research. Emerging disciplines such as Clinical Epidemiology, Molecular Biology, Clinical trials research, Observational studies, Physiology, Imaging, and Genomics and Pharmacokinetics have all contributed to making medical and health sciences depend more and more on Biostatistics. The present write-up focusses on role and use of bio-statistics in the epidemiology, bio-medical research as well as some to touch upon on-going development of new methods in response to the new challenges and problems arising in biological sciences. The attempt will be to provide a brief description on these aspects.
1.Bio-statistics as a discipline
There has been explosive growth in the development of statistical methodology over the past several decades.Research in medicine and public health has been both abeneficiary of this new methodology and a source of new problems, to the extent that statistics applied to medical research – biostatistics – can nowadays be considered adiscipline in its own right.In fact, biostatistics has become a defined branch of science that uses an intricate combination of statistics, probability,mathematics and computing to resolve problems in the biomedical sciences. Because research questions in biology and medicine are diverse, biostatistics has expanded its domain to include any quantitative, not just statistical, model that may be used to answer these questions. As a discipline designed to yield information, biostatistics may also be considered as a (highlydeveloped) branch of medical informatics, which in turn forms part of the developing field of bioinformatics. Consequently, biostatistics draws quantitative methods from fields including statistics, operations-research, economics, and mathematics in general; and it is applied to research questions in fields such as public health (including epidemiology, nutrition, environmental health, and health services research), genomics and population genetics, medicine, and ecology.
The important role that biostatistics and biostatisticians play in the field of medical research has always been widely recognized by the biomedical community, and today statistics applied to medicine can be considered a successful model for the introduction of statistics into scientific practice. The importance of bio-statisticians/ Biostatistics in the biomedical field can be well appreciated as there being called upon to render their services asadvisors in the prestigious committees and Journals. In addition, specialized statistical journals like Biostatistics, Biometrics, Biometrika,and many other journals relating to biostatistics are considered highly prestigious with in statistics.1-2
2) Types of research investigations in biomedical field
Careful study design forms the foundation of quality Bio-medical research. In the last few decades, newer and newer concepts and statistical methods have been developed and are being employed for the design and analysis of data in biomedical studies. Development has been on the design of studies such as case-control studies, cohort studies clinical trials and survival studies. A recent development has been on the application of epidemiologic principles and methods to the design, conduct and analysis of clinical trials whose detailed applications are provided in the undermentioned paragraphs. Research investigations in bio-medical filed are classified into two types: observational and experimental. Some of the important questions which arise during the design of statistical design of investigations are selection of subjects on which observations are to be made, allocation of subjects among different groups, sample size for the study, procedure for randomization in experimental studies, selection of controls etc. The issues relating to the planning of investigations in medical research with emphasis on statistical design has been published elsewhere.3-5
3.Why is statistics necessary in bio-medical field?
Empirical research in any field is incomplete without appropriate inferences and bio-medical research is no exception to this. Use of appropriate statistical techniques are needed both at the designing of various bio-medical research studies and in the interpretation of results. As we have noted, the growth of quantitative methodsin the biomedical sciences (bio-chemical, physiological, clinical parameters or evidenced based medicine) has made biostatistics a key component in many research areas. Medicine is a science with chance playing a very significant role. Statistics as science help to quantify the contribution of chance and as an art helps individual clinician make valid diagnostic, prognostic or therapeutic decisions. It also helps health programme managersand policy planners to plan monitor and evaluate public health initiatives and the quality of health care delivered to the population through several health indicators. A health indicator can be used to describe one or more aspects of the health of an individual or population (quality, quantity and time) and also are used to define public health problems at a particular point in time, to indicate change over time in the level of the health of a population or individual, to define differences in the health of populations, and to assess the extent to which the objectives of a program are being reached. Similarly, several validity measures such as sensitivity, specificity, positive and negative predictive value, are employed to describe the quality and usefulness of a diagnostic test or find out efficiency of a marker in the diagnosis of a disease3 . To highlight how bio-medical research depend on statistics as a fundamental tool in the achievement of the goals two major fields of medical research, namely epidemiology and clinical trials have been considered.
3.1. Epidemiology and Biostatistics
Epidemiology is the study of how often diseases occur,and why, in different groups of people. Epidemiological information is used to plan and evaluate strategies to prevent illness, and serves as a guide for the management of patients in whom disease has already developed. The connection between biostatistics and epidemiology has always been close. The early epidemiologists werephysicians basically interested to understand interested the way in which diseases occur in populations, their causes, and their relationships with different medical and non-medical factors. The problems tackled by these pioneers were not only confined to the study of epidemics, noncommunicable diseases such as relationship of smoking with lung cancer but also extended to the evaluation of therapies. Many were skilled in quantitative reasoning and were knowledgeable about the statistical methods of their day. Then, from the 1930s on, epidemiology began to turn its attention to the study of chronic diseases. It became impracticable to use the same prospective research strategies that had been so obviously appropriate in the study of infectious diseases. And here it was statisticians,primarily Cornfield and Mantel, who provided a rationale for approximately valid inference based on case-control data. Biostatisticians became more involved in elaborating on the conditions for valid inference, with concerns about bias due to possible confounding factors. Further more, they began exploring other issues related to epidemiologic research, such as models–called doseresponse models– for evaluating the effects of possible risk factors for disease. These effects are quantified by measures of association such as the odds ratio or relative risk, i.e. probabilistic concepts that need to be estimated appropriately, according to the type of study (case-control, crosssectional, or cohort) used for each particular research project. The variety of statistical methods required in epidemiology is immense, and has led to the appearance of numerous books dealing with applications of statistics in epidemiological contexts.6-9
3.2 Clinical trials and Biostatistics
Clinical trials are an essential part of the medical research process. Through these clinical trials, scientific discovery canlead to better ways of preventing, detecting, and treating diseases and medical conditions. Clinical trials are studies performed with human subjects to test new drugs or combinations of drugs, new approaches to surgery or radiotherapy, or new procedures to improve disease diagnosis or patient quality of life. Most hospitals now take part in clinical trials, which are only begun after laboratory studies have indicated that the new treatment or procedure is apparently safe, and that it has the potential to work better than existing options.Recent years have seen a major increase in the importance of statistics in the field of drug development. Statistics plays an essential part at all stages of the clinical trial, from planning, through conduct and interim analysis, to final analysis and reporting10-11. The statistician will typically devise the randomization schedules, advise on sample size, specify criteria for measuring treatment differences, and analyse response rates. The statistician will generally also be the link with the Independent Data Monitoring Committee.Several emerging and recurrent issues relating to the drug development process merit particular mention.
Recurrent issues include the continuing development ofstatistical methods for handling subgroups in the design and analysis of clinical trials; alternatives to the “intention- to-treat’ analysis in the presence of non-compliance in randomized trials; methodologies to address the multiplicities resulting from a variety of sources, inherent in the drug development process; and methods to assure data integrity. These issues pose a continuing challenge to the international community of statisticians involved in drug development. Moreover, the involvement of statisticians with different perspectives continues to enrich the field and contributes to improvements in public health. The important methodological contributions being made by biostatisticians to clinical trials research has led to the recent creation of a specific journal, Pharmaceutical Statistics having appeared only in 2002, it is already in the JCR ranking for Statistics and Probability.
4) Advanced statistical areas of interest in bio-medical field
In addition to routine descriptive and inferential statistics, the areas of statistics that have most influenced medical statistics in recent yearshave been generalized linear models (including multiple linear regression, linear discriminant analysis), variables with time related events, categorical data analysis, survival analysis, and Bayesian methods (in diagnostic, epidemiological and clinical trials contexts). The statistical methods of multiple regression or linear discriminant analysis methods are used in bio-medical field to make a prediction of dependent variable with the help of other independent variables/ characteristics or to separates the individuals in to two or more classes of objects or events depending on disease status. The above procedures have helpedfor dimensionality reductionas well as for classification of persons in to diseased or non diseased or in to various categories of diseases status. Meta-analysis,as a tool for evidence-based medicine, decision analysis based on Bayesian perspectivehas likewiseattracted considerable attention in recent year. In addition to this, for classifying the persons between diseased nor diseased.7-13
7. Modeling-approach in epidemiological research/bio-medical filed - generalized linear models
Modeling of health and disease process has been a complex phenomenon. Several models have been employed for the analysis and interpretation of data in the biological field. In the forgoing sections, it is proposed to describe three different types of general linear models which have been extensively employed as multivariate procedures in biomedical field viz. (i) Age-period cohort models, (ii) Logistic regression model and (iii) Survival analysis.
7.1) Importance of time-related analysis
Data on cancer incidence/mortality rates from population-based registries (which collect information on all cancer cases in defined areas) provide information on geographical and temporal variation in cancer risk by personal characteristics such as age, sex and racial or ethnic groups. “Time”, the third element of epidemiological descriptor provide information on geographical areas and they form as the basic elements of information from which to judge how successful the measures are taken in reducing the burden of cancer. Changes in cancer pattern with passage of time are of vital interest in cancer control activities. The time related confounders that have been most frequently considered include age at risk, calendar year and birth cohort effect. The trend analysis helps to understand the question such as how cancer risk has been changing, why and what is likely to happen in future. Cancer trend analysis is important information for the public health and health care planning. Trend analysis offers clues as to the understanding of causes of the disease and wide variation in the frequency around different geographical areas. Cancer incidence/mortality trends also provide a prediction of future cancer patterns, which will be guidance for drawing future public health policy. Modelling of data through age, birth cohort and calendar time period are the appropriate techniques for analyzing trends in cancer incidence/mortality data. These models are called as “age-period-cohort (ACP) models. These models are based on Poisson distribution regression models.14
The application of the above modelling procedure has been employed to estimate the trends incidence of common cancers utilizing the data of the Indian Population Based Cancer Registries for the past twenty five years. The findings indicated that the some of the cancers such breast, ovary, corpus and uteri were increasing at almost 1-2% per annum and the same increase were also noted in younger age group of women also.15-18
7.2) Modelling of the data in case of binary outcome event:
When the dependent variable (outcome variable) happens to be binary in nature viz. an event occurring or not, taking values unity or zero, the assumption necessary for fitting multiple linear regression model of the type Y=α+Σk i =1 ßXi is violatedas it is unreasonable to assume that distribution of errors as normal. For such data, instead of multiple linear regression analysis, multiple logistic regression analysis (LR) analysis is carried out as a multivariate procedure to identify the independent predictors of the outcome variable. The main difference between LR and multiple linear regression model is that instead of using the dependent variable as such, we use a model based on logit transformation of the dependent variable to satisfy the needed assumptions. Thus in LR model we predict the proportion of subjects (P) with a particular characteristic or equivalently, the probability with characteristics for any combination of the explanatory variables.8,19-20
The model relates a dichotomous outcome variable to a series of “k” known or suspected factors (regression variables) and possible confounding and effect modification variables. The collection of k regression variables or risk factors is called as covariates or explanatory variables. The unknown parameters in the model α, and ßi are estimated using the method of maximum likelihood estimation. The likelihood inference typically precedes by fitting hierarchy of models each one containing the last variable. The hypothesis testing is done through likelihood ratio test or test based on Wald statistics.The application of this modelling procedure has been employed to identify the independent risk factors associated with disease or an adverse outcome event. In a study to analyze the effect of maternal and perinatal outcome in varying degrees of anaemia logistic regression method was employed. The findings indicated that mild anemia fared best in maternal and perinatal outcome. Severe anaemia was associated with increased low birth weight babies, induction rates, operative deliveries and prolonged labour.21
7.3) Studies on survival analysis
The analysis of lifetime data is important in understanding the relationship between time and occurrence of vital and health related events. Time-to-event data is frequently encountered for analysis in bio-medical field. Such analysis is called as "survival analysis". In follow-up/ survival studies, the outcome variable is the time elapsed between the entry of a subject into the study and the occurrence of an event is related to treatment. The event of interest (development of a disease, death) has been referred to failure and the outcome variable as the survival time. In oncology, for example, interest typically center’s on the patient’s time of survival following a surgical intervention. The analysis of this type of survival experiment is complicated by issues of censoring and truncation. Censoring occurs when we do not fully observe the patient’s survival, due to death unrelated to the cancer under study, or disappearance from the study for some reason. The other factor is truncation, which basically occurs when some patients can’t be observed for some reasons related to the survival itself. A common example of this is in HIV/AIDS studies of the incubation period (i.e. time from infection to disease). The follow-up starts when the HIV virus is detected and the moment of infection is retrospectively ascertained. Several survival parametric models such as Exponential and Wiebull distributions were introduced to model the survival experience/follow-up data analysis of homogeneous populations incorporating the censoring schemes. The distribution of survival times must be known to apply these models. However, when the distribution of survival is not known, the non-parametric method of KaplanMeier curve developed in 1959 has been a wellknown estimator of the survival function, and it is extensivelyused in epidemiological and clinical research.22-26
In order to take into account diversity of situations, which were encountered in practice, Cox in 1972 developed a modelling procedure termed as Cox-proportional hazards modelunder a very rigorous theoretical backup. The classical proportional hazards model of Cox (1972) is also widely used whenever the goal is to study how covariates affect survival. This model is an important tool in the follow-up/survival studies for modelling the effect of risk factors/prognostic factors when the outcome of interest occurs with time. In the model, the hazard for an individual is a part of the product of a common baseline hazard and a function of set of risk factors. By applying the above modelling procedure the independent risk factors associated with the development of precancerous lesions of cancer of cervix was evaluated.27 Similarly, in an another study the treatment effectiveness for curing of a gastro intestinal bleeding was evaluated which employed an experimental design.28
However, when the assumption of proportionality does not satisfy, then a classical approach for the analysisof data of this type is the time-dependent Cox regression model (TDCM). Advantages of Cox’s regression model include its easy interpretability and its availability in the majority of statistical packages.
7.4 New issues in survival analysis
A generalization of the survival process arises when survival is the ultimate outcome but intermediate states are identified. In this situation, a sequence of events is observed, leading to more than one observation per individual. Intermediate states might be based on categorical timedependent covariates such as transplantation, clinical symptoms (e.g. bleeding episodes),or a complication in the course of the illness (e.g. metastasis), or alternatively on biological markers (like CD4T-lymphocyte levels).
In the 1990s, so-called multi-state models (MSMs) became available: these offer a better understanding of the disease process, leading to a better knowledge of how the time dependent covariate affects the evolution of the disease. These modern models have several advantages over Cox’s regression model. They offer a better understanding of the disease process, providing the hazard for movement out ofone state into another (i.e. transition intensities), as well as many other types of information, including the mean time of sojourn in each state, and survival rates for each state. Covariates on transition intensities can also explain differences in the course of the illness among subjects in the population. Notably, MSMs can reveal how different covariates affect different transitions, something that is not possible with other models like the TDCM. In fact, it is very unlikely that the risk of death in patients who have received different treatments will be the same. Furthermore,the prognostic factors associated with the risk of deathmay differ depending, e.g., on the treatment received.A considerable literature is nowadays available on the analysis of MSMs.29-33
8.Some recurrent and emergingissues in biostatistics
Modern biostatistics presents a number of challenges interms of both the continuing development of classical techniques and the creation of new techniques to resolve new problems. We thenturn our attention to various emerging fields that meritfurther research by biostatisticians: specifically bio informatics, spatial statistics, neural networks, and functional data analysis, big data analysis.34-43
8.1: Statistical methods in bioinformatics
A very rapidly emerging influence on biostatistics is the on-going revolution in molecular biology. Molecular biology is now evolving towards information science, and is energizing as a dynamic new discipline of computational biology, sometimes referred to as bio-informatics. Bioinformatics merges recent advances in molecular biology and genetics with advanced statistics and computer science. The goal is increased understanding of the complex web of interactions linking the individual components of a living cell to the integrated behaviour of the entire organism. The availability of large molecular databases and the decodingof the human genome may allow a scientist to plan an experiment and immediately obtain the relevant data from the available databases. This is an area in which statistical scientists can make very important contributions. Several biostatistics departments (mainly in the U.S.) have already been renamed as “Biostatistics and Bioinformatics”.34-35
8.2: Spatial statistical methods in health studies
The analysis of the geographical distribution of the incidenceof disease and its relationship to potential risk-factors has an important role to play in various kinds ofpublic health and epidemiological study. This general areais referred to as “geographical epidemiology”, and fourbroad areas of statistical interest can be identified: (a) Disease mapping aims to produce a map of thetrue underlying geographical distribution of disease incidence, given “noisy” observed data on disease rates.
(b) Ecological studies are concerned with associations between the observed incidence of disease andpotential risk factors, as measured on groups rather than individuals, the groups typically being definedby geographical area. Such studies are valuable in investigating the aetiology of disease, and may helpto identify future lines of research, and possibly preventativemeasures.
(c) Disease clustering studies focus on identifying geographical areas with a significantly elevated risk of disease, or on assessing the evidence of elevated riskaround putative sources of hazard. Uses include the targeting of follow-up studies to as certain reasons for observed clustering in disease occurrence, or the investigation of control measures where the aetiology of observed clustering has been established. (d) Environmental assessment and monitoring is concerned with as certaining the spatial distribution of environmental factors relevant to health, and exposureto them, so as to establish necessary controls ortake preventative action. Given the breadth and importance of the concerns ingeographical epidemiology, it is not surprising that there has been considerable interest in this area in recent years.36-38
8.3. Neural networks in medicine
Neural networks (NN) approaches in medicine have attracted many researchers, and these approaches have been implemented in several biomedical applications, including diagnostic systems, bio chemical analysis, image analysis, and drug development. Neural networks, which simulate the function of human neuron networks, have potentially useful implementations inmany applications domains. Unlike human decisionmakers, NNs are of course unaffected by factors like fatigue, working conditions and emotional state. NNs have been applied in various areas of medicine: theyare widely used in diagnostic systems, for the detectionof cancer and heart problems, and for the analysis of diverse types of medical image (including tumour detectionin ultra-sonograms, classification of chest x-rays, tissueand vessel classification in magnetic resonance images estimation of skeletal age from x-ray images, and assessmentof brain maturation). NNs are used experimentally to model the human cardiovascular system: diagnosis can beachieved by building a model of the cardiovascular system of an individual and comparing it with the realtime physiological measurements taken from that patient. NNs are also used as tools in the development of drugs for treating cancer and AIDS. Neural networks are increasingly being seen as an extension to general statistical methodology, to be given full consideration along side classical and modern statistical methods.39-41
8.4. Functional data analysis and medicine
In recent years, because of technological progress, many scientific fields in which applied statistics is involved arenow measuring and recording continuous (i.e. functional) data. Notably, many modern apparatuses allow biomedical researchers to collect samples of functional data (mainly as curves, though also as images). Since functional data is presented in curve form, it isnatural to use the curve as the basic unit in functional data analysis. Functional data tend to involve a large number of repeated measurements per subject, and these measurements are usually recorded at the same (often equally spaced) time points for all subjects, and with the same high sampling rate. Functionally, of these curves, such as derivatives and locations and values of extremes, are sometimes also of interest. This situation is very commonin areas of basic medicine like endocrinology, for examplein studies of hormone levels after different drug doses; Orin neuroscience, for example in studies to estimate thefiring rate of a population of neurons, in which the unit ofstudy is the firing curve of each individual neuron. Anotherexample is in the study of growth curves where more thanone characteristic of growth is observed, e.g. height andlung function.40
The aims of functional data analysis are usually of an exploratory nature to represent and display data in orderto highlight interesting characteristics, perhaps as inputfor further analysis. However, there may be other aims, including estimation of individual curves from noisy data, characterization of homogeneity and patterns of variability among curves, and assessment of the relationships of shapes of curves to covariates. In spite of the important recent contributions in functional data analysis, the development of new tools to deal with functional data represents a significant challenge for thestatistical community. Apart from the books already cited, a good starting point for thinking about new lines of statistical research and possible applications may be the special issue on functional data due to appear soon (2007) in the journal Computational Statistics and Data Analysis.
9. New statistical methods which are likely to play a key role in biomedical research over coming years:
The following new statistical methods are likely to play a key role inbiomedical research over coming years: (i) bootstrap (another computer-intensive methods); (ii) Bayesian methods); (iii) generalized additive models;(iv) classification and regression trees (CART); (v) modelsfor longitudinal data (general estimating equations); and (vi)models for hierarchical data, (vii) big data analysis.
10.Training biostatisticians
For the successful application of statistics in biomedical research, requires professionals with a high-level mathematical training and appropriate training of bio statisticians in the relevant biomedical disciplines, such as epidemiology, clinical trials, molecular biology, genetics, and neuroscience, bio informatics, basic biology, as well as in operation research methods, communication and leadership skills. The demand on leadership is likely to be even greater than in the past, given the central role that biostatistics and bio informatics now play in biomedical research. Thus, a training programme in biostatistics must by necessitybe inter disciplinary, connecting statistics training per se to an understanding of the basics of biomedical research. A modern training programme ofthis type, with an emphasis on bioinformatics, statistical genetics and computational biology, would profit from trainees spending time in biomedical laboratories to gainfirst-hand experience and insight into the nature of the real problems faced by these researchers. A major goal must be to train students to become independent researchers advancing the field of statistical research andits application to bio medical research, both basic and clinical; and at the same time to be team workers intimately involved in the design and data analysis of collaborative bio medical projects. Since many bio medical problems will likely require the development of new statistical methods, students should be capable of critically reading the theoretical and methodological literature in statistics, and of developing new methods as appropriate for the problems that they encounter.44
The traditional component of biomedical courses will probably focus on areas of mathematical statistics including probability theory, inference, re-sampling methods (e.g. bootstrap), linear regression, analysis of variance, generalized linear models, survival analysis (including multistate models), nonparametric methods, and data analysis. In addition, new methodologies likes patial statistics, neural networks, smoothingregression methods (such as generalized additive models) and operations research are strongly recommended. The decision technologies, tools and theories of operations research and management Sciences have long been applied to a wide range of issues and problems within health care.
Modern health research involves increasingly sophisticated statistical tools and computerized systems for data management and analysis. During the past few years tremendous amount of software has been made available to support statistical computing requirements for biomedical research. Bio-statisticians have to be extremely familiar with various statistical software packages such as STATA, R,SAS, SPSS etc.
Supporting File
References
1. Altman DG and Goodman S, “Transfer of technology from statistical journals to the biomedical literature: Past trends and future predictions” Journal of American Medical Association, 1994, 272-329.
2. Suarez C C and Manteiga,WG, ARBOR Ciencia, Pensamiento y Cultura CLXXXIII 725 mayo-junio (2007) 353-361 ISSN: 0210-1963, STATISTICS 2007.
3. Hennekens CH. Buring IE. Design strategies in epidemiologic research. In: Mayrent SL (ed). Epidemiology in medicine. Boston: Little, Brown and Co., 1987: 16-20.
4. Mathew A and Murthy NS. A step towards quality medical research. National Medical J India. 11, 6, 283-286, 1998.
5. Prentice RL, Statistical methods and challenges in Epidemiology and Biomedical Research, Handbook of statistics, Elsevier, 2008,
6. NSN Rao and NS Murthy, Applied statistical methods in Health Sciences”, JAYPEE Brothers Medical Publishers (P) LTD, 246 pages, NewDelhi, 2008, second edition, 2010.
7. Armitage P. Statistical methods in medical research. Oxford: Blackwell Scientific Publications, 1983:167-88. 3
8. Breslow NE and Day NE, Statistical methods in cancer research. Vol 1, -The analysis of case-control studies. International Agency for Research on Cancer, Lyon, 1980.
9. Breslow NE and Day NE, Statistical methods in cancer research. Vol 2,-The analysis of cohort studies. International Agency for Research on Cancer, Lyon, 1987.
10. Murthy NS, Mathew A, Sharma JB: Design of research studies in clinical Obstetrics and Gynaecology: Obtetrics& Gynaecology Today; 6, 7(1), 379-386, 2001.
11. Peto R, Pike MC, Armitage P, Breslow NE, Cox DR, Howard SV, Mantel N, McPherson K, Peto J, Smith PG. Design and analysis of randomized clinical trials requiring prolonged observation of each patient. Part II: Analysis and Examples. British Journal of Cancer 1977;35:1-39.
12. Armitage P, Berry G. Statistical Methods in Medical Research (3rd edition). Blackwell 1994.
13. Houwelingen (van) HC. The future of Biostatistics: Expecting the unexpected”. Statistics in Medicine, 16, 2773-2784, 1997
14. Clayton DG and Schifflers, E, Models for temporal variations in cancer rates I and II, Stat. Med6, 1987449-467, 469-481
15. Murthy NS, Burra U, Chaudhry K, and Saxena S: Changing trends in incidence of breast cancer-Indian Scenario. Indian Journal of Cancer, 2009.
16. Murthy NS, Shalini S, Suman G, Pruthvish P, and Mathew A; Changing trends in incidence of Ovarian Cancer – Indian Scenario, APJCP, 10, 2009, 1025-1030
17 Murthy NS,Shalini P, Nandakumar BS, Suman G, Pruthvish S and Mathew A; Estimation of trends in incidence of cancer of corpus uteri – Indian Scenario, European Journal of Cancer Prevention 2010, 20, 1, 25–32
18. Shalini C N, Murthy NS, Shalini S, Pruthvish S, Mathew A, Trends in Rectal Cancer Incidence - Indian Scenario,Asian Pacific J Cancer Prev, 12, 2077-2082, 2012
19. Schlesselman JJ. Case-Control Studies. New York: Oxford University Press 1982.
20. Murthy NS, Juneja A andSharma S. Modelling strategies for epidemiological process with special reference to logistic regression. Ind. Jour. Prev. and Soc. Med.35 (3&4), 136-145. July-Dec. 2004.
21. Malhotra M, Sharma JB, Batra S, Sharma S, Murthy N.S, Arora R; Maternall and perinatal outcome in varying degrees of anaemia; Inter. J Gynae. & Obstet. 79, 2, 93-100, 2002
22. Kaplan EL, Meier P. Nonparametric estimation from incomplete observations. Journal of the American Statistical Association 1958;53:457- 481
. 23. Cox DR. Regression models and life tables. Journal of the Royal Statistical Society 1972;B34:187-220.
24.. Kalbfleisch JD, Prentice RL. Statistical Analysis of Failure Time Data. New York: Wiley 1980.
25. Mathew A, Pandey M and Murthy NS. Survival analysis, caveates and pitfalls. Eur J Surgical Oncology, 25, 321-329, 1999.
26. Muthry NS, Mathew A, Yoele BB, and Happanen N: Cohort data analysis: Is logistic regression or Cox proportional hazard model or Poisson regression model? Ever green problems in Epidemiology (Ed) LeenaTenkanen, Publication 3, School of Public Health, University of Tampere, 1999.
27. Murthy NS, Sehgal A, Satyanarayana L, Das DK, Singh V., Gupta MM and Luthra UK. Risk factors related to biological behaviour of precancerous lesions of uterine cervix. Br. Jour. of Cancer. 61, 1990.
28. Sarin SK, Lamba GS, Kumar M, Mishra A and Murthy NS. A comparison of endoscopic ligation and propronolol for the primary prevetioin of varical bleeding, New Engl J Medicine, 340, 13, 988-993, 1999
29. Klien J P and and Moeshberger M L, Survival analysis techniques for censored Truncated data, Springer Science Business India 2006
30. Moeshberger M L and Klien J P, Statistical methods for dependent competing risks, Life data analysis, 1995,Springer
31. Klien J P and and Moeshberger M L, Independent or dependent competing risks: Does it make difference. Tyler and Francis , 1987
32. Andersen, P. K., Borgan, O., Gill, R. D. and Keiding, N. (1993): Statistical Models Based on Counting Processes, Springer, New York.
33. Hougaard, P. (2000): Analysis of Multivariate Survival Data, Springer, New York.
34. Vapnik V, The nature of Statistical learning Theory, Springer-verlag, 1995
35. Ewens, W. J. and Grant, G. (2005): Statistical Methods in Bioinformatics (Statistics for Biology and Health), Springer, New York.
36. Cressie N. 1993 . Statistics for Spatial Data. John Wiley & Sons New York,
37. Spatial Prediction and Kriging 1993, Wiley Series in probability and
38. Statistics for Spatial Data Wiley Serie4s, in Probability and Statistics.
39. Ripley B D (1996) . Pattern Recognition and Neural Network, Cambridge University Press
40. Radhika Kl, George PS, Mathew BS, Mathew A: Comparison of artificial neural network with logistic regression as classification models for prediction of breast cancer patients’ outcome. International conference in Epidemiology; 2012.
41. Ripley B D “Statistical aspects of neural networks”. In Barndorf-Nielson O E, Jensen, J L, Kendall W S (Eds). Networks and ChaosStatistical and Probabilitic aspects, London, Chaman & Hill Press., 1993.
42. Ramsay J and Silverman B Applied Functional data Analysis, 2nd Edition, Springer, New York.
43. Radhika Kunnavil1, Murthy NS , Healthcare Data Utilization for the Betterment of Mankind - An Overview of Big Data Concept in Healthcare, International Journal of Healthcare Education & Medical Informatics, Volume 5, Issue 2 - 2018, Pg. No. 14-17
44. DeMets, D. L., Stormo, G., Boehnke, M., Louis, T. A., Taylor, J. and Dixon, D. (2006): “Training of the next generation of biostatisticians”, Statistics in Medicine 2006, 25, 3415-3429.,