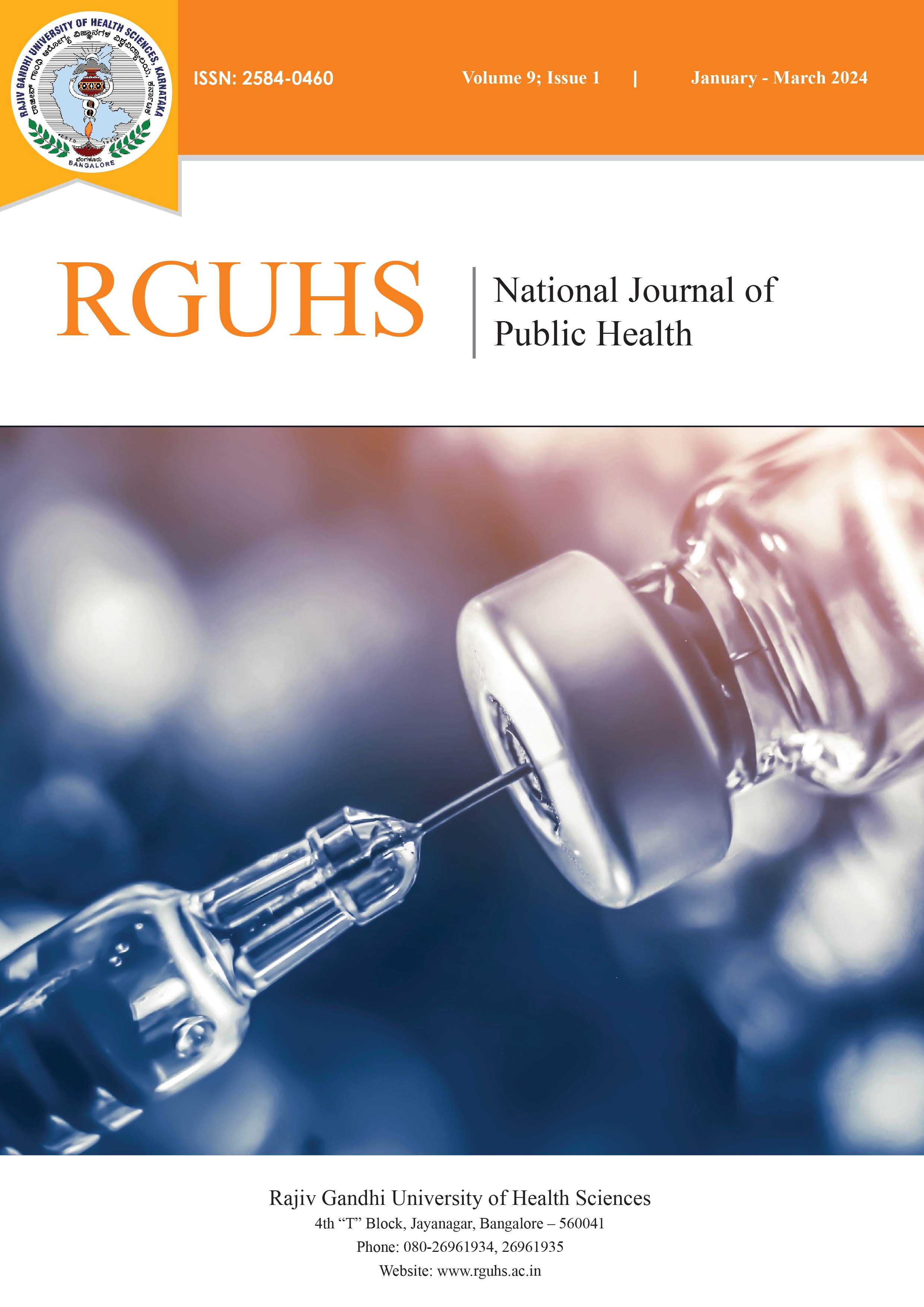
RGUHS Nat. J. Pub. Heal. Sci Vol No: 9 Issue No: 1 eISSN: 2584-0460 pISSN:
Gangaboraiah1
1: Professor of Statistics
Address for correspondence:
Mr. Gangaboraiah
Professor of Statistics,
Rajiv Gandhi Institute of Public Health
RGUHS), Bangalore, India.
E-mail: nisargboraiah@gmail.com

Abstract
Research and Statistics are inseparable. The major steps in the research process are Research question, Aim and Objectives, Research design, Statistical hypotheses, Methodology and Analysis of data.
Keywords
Downloads
-
1FullTextPDF
Article
Introduction:
Research is dynamic, more so in medical research. Research and Statistics are inseparable. As quote goes “Medicine without Statistics is like a ship without compass” – J E Park & K Park. In addition to the above quote, it can also be said that “in research, Epidemiology is like a heart and Statistics is like a spine”. To understand how statistical methods play a significant role in medical research the following schematic diagram on research process is to be understood.
The major steps in the research process are Research question, Aim and Objectives, Research design, Statistical hypotheses, Methodology and Analysis of data. Among these, the following are to be paid utmost importance in the preparation of research protocol.
Research question: The role of statistics starts from here: in identifying the suitable target population from where the optimum number of representative samples will be selected to collect the reliable and consistent data to answer the research question. The researcher should pay a careful attention towards PICO and FINGERS, which constitute 25% of planning of conduct of research.
Aim and Objectives: In any research, there will always be one Aim and many Objectives. The Aim addresses the global solution to the research question which is achieved through suitably framed objectives. Objectives are of two types viz., Primary objective and Secondary objective. For all practical purposes, the primary objective is very important as it relates to the main outcome of the study, in testing the research hypothesis (if any to be tested), the sample size calculation, and the power of the study. The secondary objectives can serve as axillary interest of the selected research problem. While framing objectives, attention must be paid towards SMART, in which measurability is most important. It uses measurable action verbs like ‘to describe’, ‘to assess’, ‘to find out’, ‘to correlate’, to estimate’, ‘to determine’, etc. The researcher should never ever use action verbs like ‘to study’, ‘to know’, ‘to see’, ‘to observe’, ‘to believe’, ‘to define’, etc which are not measurable statistically. Further, it is also equally important to list the variables to be used generate the data to measure each of the objectives.
Research Design: The research design forms the heart of the research problem. It is said that ‘a strong research design requires the usual statistical methods to analyze the data, but if the research design is weak, then what ever may be the sophisticated statistical methods chosen to analyze the data may not be able to take care the gap created by poor research design’. Based on the problem statement any of the following epidemiological designs can be selected.
Besides epidemiological designs, the following are some of the statistical designs used in case of experimental studies, which should also be specified at the time of preparing research protocol.
(a) One group before-and-after design
(b) Two groups after only concurrent parallel design
(c) Two groups before-and-after concurrent parallel design
(d) More than two groups after only concurrent parallel design
(e) More than two groups before-and-after concurrent parallel design
In order to use the above designs, the researcher should also verify the following principles of experimental designs viz.,
(a) Randomization
(b) Replication
(c) Local Control
In case of not being able to go for random allocation of study subjects, then such study design will be referred as Quasi experimental design’, which will need a specific mention in the protocol. The purpose of knowing statistical designs helps the researcher to know what statistical tests should be applied to arrive at the inference.
2. Statistical hypotheses: Another important step in research process is to specify what type of ‘research hypothesis’ the researcher is intended to test (must be specified in the protocol). Stating research hypothesis in the protocol plays a vital role in the calculation of sample size, because one-tailed hypothesis need less number of samples, whereas two- tailed hypothesis requires more samples. Suppose the researcher may wishes for two-tailed hypothesis, but the sample size calculated is for one-tailed, then it might lead to insufficient data to prove what the researcher is intended to prove. This particularly is very important in clinical trials, where to prove clinical significance statistically, optimum size of the hour will be needed. Further, research hypothesis is also directly related to ‘power of the study’.
3. Methodology: In addition to sample size calculation, importance should be given to inclusion and exclusion criteria. A properly framed inclusion and exclusion criteria takes care of the issues like ‘confounders’, yet another issue the researcher has to address in the study. The next step is to decide what sampling techniques should be used for collecting the data. For a better generalization of the results, it is always better to adopt ‘probability sampling techniques’. In inevitable situations, non-probability sampling techniques may be used, but they will serve only as a baseline data and once again further studies need to be conducted to generalize such results. As for methods of data collection is concerned, one should decide between either oral interview technique or self-administered questionnaire. Even though, the telephonic conversation, postal, and internet methods are also can be made use, they may not ensure consistency in the data and may possibly lead to Berkosonian bias. However, in special cases, only for follow up data collection telephonic conversation method may be used ensuring free from communication barriers. For more reliable and consistency in the data, it always better to prepare the questionnaire
using ‘Item analysis’, so that the first 27.5% ‘very easy level’ and 27.5% ‘very difficult level’ questions can be removed, resulting in the middle 45% questions which can be answered by all category of responders.
Analysis of data: This is one of the crucial step in research process. Suitable statistical methods are to be chosen to analyze and present the results. Even though many statistical software’s are available to analyze the collected data, but research should have good amount of knowledge on choosing right method for analysis. Some of the reputed paid software’s available are ‘SPSS’, ‘SAS’, ‘STATA’, ‘Sys Stat’. There are many open source software’s viz., ‘R’, ‘Python’, ‘Epi info’, ‘PSPP’. Of these, ‘R’ and “Python’ requires some basic training to use, because they are based on coding. Although ‘Epi info’ requires some hands on training to work, ‘PSPP’ is menu driven like ‘SPSS’.
The first step in data analysis is to identify the type of variables measured and any possible relationship between them. The second step is based on the research design used in the research. The analysis may be carried out as follows:
If the study design is descriptive and the variable(s) measured is/are qualitative/ categorical, then construct a frequency table, express the result in percentages and represent the results graphically if necessary. In case, the sample proportion is estimated, then find the standard error of proportion and 100(1-α) % confidence interval for population proportion. This helps the researcher to convey with what confidence the parameter of the population lie within the sample estimates, which is the ultimate goal of conducting studies based on samples
If the study design is descriptive and the variable(s) measured is/are quantitative, in addition to express the data categorical in the form of frequency table with percentages and graphs wherever necessary. The emphasis should be given to calculate Mean and SD along with standard error and 100(1-α) % confidence interval for population mean or Median and inter quartile range (IQR). It is very important to remember in case of analysis of data on quantitative variable that, the data should be checked for normality assumption. If the data is distributed near normal, better to use Mean ± SD to describe the data, otherwise use Median and IQR for skewed data. In case of highly skewed data, the outliers are to be properly treated (either to retain or impute). The Box-and-Whisker plot helps to a great extent to tackling the outliers.
If the study design is comparative and the variables measured is qualitative/ categorical, then there are two ways to analyze:
(a) Suppose the statistical estimate is proportions, then the difference between two proportions are to be tested using Standard normal distribution test (Z – test) for specified α-value, however, based on the test-statistic Z, P-value has to be calculated. (No software has this facility to calculate directly Z – value).
(b) Suppose the independence (or no association) between two categorical variables are to be tested, then Pearson’s Chi-square test is the better choice. Caution: In case, the expected frequency in any cell is < 5 in a 2 x 2 contingency table, then apply Fisher’s exact probability test (though Yate’s correction is an alternate choice, it gives only approximate value). But, for a m x n contingency table having expected frequency < 5, modify the table by merging either rows or columns meaningfully and apply the Chisquare test. The degrees of freedom will be reduced accordingly.
If the study design is comparative and the variables measured are quantitative, then the following cases are to be considered to analyze data statistically:
(a) Suppose there are two independent groups and the variable measured is one, then by subjecting to the normality assumption verification using Kolmogorov-Smirnov test or Shapiro-Wilk test; the Student’s independent two-sample (unpaired) t-test can be applied to test the difference between two population means. The standard error of difference between two means and 100(1-α) % confidence interval should also be computed to make the results more meaningful and acceptable. The second assumption of equality of variances can be tested using Leven’s F – test. In case, the variances are not equal, still continue to apply unpaired t-test (which is called as Welch t-test), but the degrees of freedom will be reduced. In case if the normality assumption do not fulfill, then MannWhitney U – test (a non-parametric test) should applied
(b) In case of single variable but the data are recorded at two different time points in the same individuals, it forms a related (paired) observation. Here owing to normality assumption verification, Student’s paired t-test should be applied. The standard error for difference between before and after observations as well as 100(1-α) % confidence interval should be computed. One important note here is that, the paired observations are related (dependent) observations and hence either Pearson’s correlation or Spearman’s rank correlation has to be computed to know the extent of relation between the two related measurements. In the eventuality, if normality assumption does not fulfill, then apply Wilcoxon signed rank sum test (non-parametric test).
(c) If one variable is measured among
(i) more than two independent groups, then subject to normality assumption verification, apply one-way analysis of variance (ANOVA) for testing equality of k (say) group means against the not equal. In case, the null hypothesis is rejected, continue with post- hoc test to examine which two group’s mean difference has contributed to not rejecting research hypothesis, a very important step in ANOVA. In case failure to fulfill normality assumption, apply Kruskal – Wallis non-parametric test.
(ii) more than two independent groups and blocks – apply two-way ANOVA. More than two independent groups, blocks, and treatments – apply three-way ANOVA (LSD). In both the cases not rejecting the research hypothesis, post-hoc test should be applied.
(iii) Suppose more than two measurements are recorded on every individual forming repeated measures, then apply repeated measures of ANOVA along post-hoc test. Failure to fulfill normality assumption apply Friedman’s nonparametric test.
If the study design is a correlational study and the variables measured are two, compute either Karl Pearson correlation coefficient or Spearman’s rank correlation coefficient depending on the nature of data. The correlation coefficient should also be subjected for testing of hypothesis using Student’s t-test and desired level of confidence interval also to be computed.
Suppose the study objective is to fit a regression model (simple or multiple linear), and to predict the outcome, first check the model assumptions:
(a) If the dependent variable is quantitatively measured and normally distributed; error terms are also normally distributed with mean zero and variance σ2 , then fit a simple or multiple linear regression model and go for prediction.
(b) If the dependent variable is categorical, it will not fulfill the normality assumption and the scatter plot will then be an S-type curve unlike the usual regression model. In such a case, fit a logistic regression model (depending on the number of categories for dependent variable, it will be called as binary or multinomial logistic regression).
P – value:
Quite often it is mistaken that, every study should have the P – value to increase its credibility so that the readers will appreciate the study and even the publisher’s will accept it publication. But what is P – value? Is it necessary to find P – value in every study?
The P – value is the strength of evidence against the null hypothesis that the true difference is zero. Corresponding to an observed value of the test – statistic, the P-value (or attained level of significance) is the lowest level of significance at which the null hypothesis would have been rejected. In other words, it is customary to fix the level of significance α (generally at 5% but rarely at 1%), and find out the attained level at which the null hypothesis may be rejected attributing the sampling variation and thus supporting alternative hypothesis which is supposed to be proved. Generally, (i) if P < 0.01%, there may be an ‘overwhelming evidence’ that supports the alternative hypothesis, (ii) if P – value lies between 1% to 5%, there may be a ‘strong evidence’ that supports the alternative hypothesis, (iii) if P – value lies between 5% to 10%, there may be a ‘weak evidence’ that supports the alternative hypothesis and (iv) ‘no evidence’ that supports the alternative hypothesis if P > 10%.
Thus the determination of P – value depends on the study design. Usually for a descriptive study, it is not possible to find the P – value, because the objective of the study is to mainly describe the occurrences in terms of percentages, Mean ± SD along with standard error and confidence interval.
Most often, the P – value will be a hyped number. Majority of research articles do not give teststatistic value and the degrees of freedom, but give only P – value which is incorrect. For a better understanding by the reader, the test – statistic value, degrees of freedom and P – value has to be given.
Now the current practice is to provide P – value along with confidence interval of the parameter. There is a relationship between the P – value and confidence interval. If the P – value is significant, the hypothesized value of the parameter(s) will not be included within the confidence interval and contrarily, if it is not significant the confidence interval includes the hypothesized value of the parameter(s). For example, in case of testing the difference between two means, ‘zero’ will not be included in the confidence interval for difference in means if P – value is significant, where as in case of odds ratio, ‘one’ will not be included in the confidence interval for odds ratio.
Conclusion
There are many more statistical methods used for analyzing the data depending on the research designs. Some of them are Survival analysis, Discriminant Analysis, Factor analysis, Classification analysis, which are advance techniques and needs special training to use them. However, all most all statistical software’s have the facility to analyze them.
Supporting Files
References
None