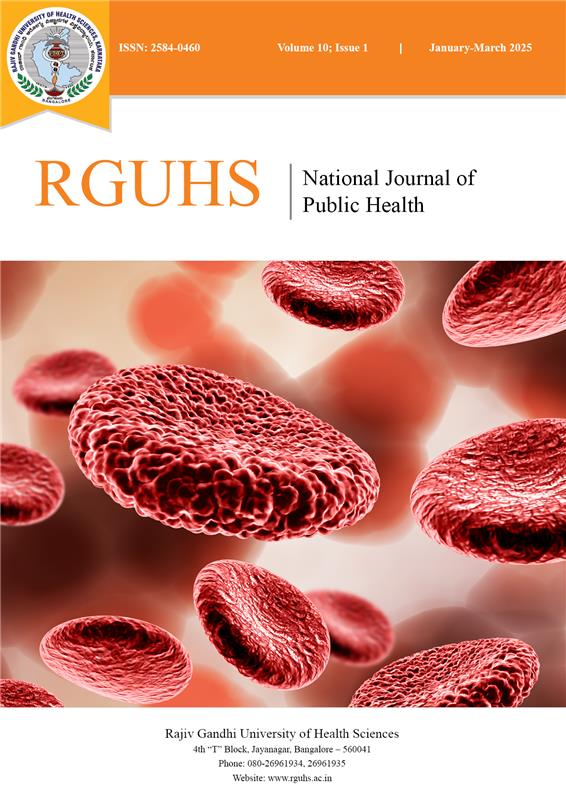
RGUHS Nat. J. Pub. Heal. Sci Vol No: 10 Issue No: 1 eISSN: 2584-0460
Dear Authors,
We invite you to watch this comprehensive video guide on the process of submitting your article online. This video will provide you with step-by-step instructions to ensure a smooth and successful submission.
Thank you for your attention and cooperation.
Rituparna Ghosh1 , Laxmi Kant Dwivedi2
1: Biostatistician, Indian Institute of Public Health, Shillong
2: Assistant Professor, International Institute for Population Sciences, Mumbai
Address for correspondence:
Laxmi Kant Dwivedi
Assistant Professor, International Institute for Population Sciences,
Mumbai
Email: rituparna.ghosh@phfi.org

Abstract
Background: Introduction of advanced technologies in health sectors have caused high, treatment and diagnostic cost to the patients. In developing countries direct out of pocket expenditure is the principle means to cope up with the great burden of health expenditure. Predominant OOP expenditure in health is indicative of a highly regressive health care system as well as poverty.1
Objective: To estimate the impact of Health insurance plans on OOP health expenditure in India.
Methodology: The study used 71st round of National Sample Survey (NSS) data from January to June 2014 on social consumption section 25.0.With multi stage sampling, 65932 households and 333104 persons were selected. OOP expenditure was calculated as the difference between total medical expenditure and the amount reimbursed for a person. Univariate and bivariate analysis was used to see the distribution of health insurance coverage. To investigate the variation in coverage at different level of sampling, multilevel logistic regression analysis was performed and intra class correlation coefficient was used.
Results: Male are more covered by any schemes of health insurance than female. People from smaller family size are more likely to have health insurance than people from bigger families. 18% urban people are under coverage while, its only 14% for rural people. 4% of the residual variance in the coverage of health insurance is explained due to the change in state while 5% is explained by the change in FSU (village). In OLS model the mean OOP health expenditure is 5058.57 Rs for insured person whereas, 6063.55 Rs for an un-insured person.
Conclusion: It shows a reduction of 5136.97 Rs in OOP expenditure for an insured person compared to an uninsured person. We can say that OLS suppress the effect of health insurance on the incidence of OOP health expenditure.
Keywords
Downloads
-
1FullTextPDF
Article
Introduction
Health is one of the most important factors at household as well as individual level for the overall wellbeing of the community. Although it is the most discussed issue world widely, in developing country like India health is considered as a secondary factor in life, especially in rural areas. Poor economic condition suppressed the need for good standard of living which leads to a poor health. In developing countries direct out of pocket expenditure is the principle means to cope up with the great burden of health expenditure.
Introduction of advanced technologies in health sectors followed by good infrastructure caused higher treatment and diagnostic cost to the patients. To bear such a high medical cost, concept of ‘distressed health care financing’ has been emerged and considered as a vital issue in recent days. When the financing of health expense is cumbersome for a household and may force the household to shift to the lower economic gradient, it is called distressed health care financing.
The broader definition of ‘distressed financing’ is adopted to include components of: (1) borrowings (with or without interest), (2) sale of assets and (3) contributions from friends and relatives (with or without repayments).1 Health Insurance is a newly emerged concept in health care financing to reduce the incidence of distressed health care financing. After 10 years NSSO 71st round came with the data on social consumption on health in India. During this gap, a number of changes have been taken place in India related to health and economy. India is world’s third largest economy and potential to grow larger in a more equitably. The second important change is the emergence of ‘health care industry’ at 15% compound annual growth rate during the global economic slowdown. Another remarkable change is the incidence of catastrophic health expenditure (CHE) is growing and now one of the leading reasons of poverty.2 out-of-pocket health expenditure can be said as catastrophic expenditure when it constitute a large part of the household expenditure and thus affect the standard of living of the people.3 A large proportion of health spending out of total household spending is responsible for cutting down other expenses. Thus, Russell (1996), explained this concept as the opportunity cost of health care spending. Rich household may sacrifice some ‘unnecessary’ cost to bear the emergency health spending but poor people have to foregone essential consumption to support OOP health expenditure.4 Individuals who are poor are most sensitive to the cost of health care. They are less likely than are those who are rich to seek care when they are ill, and this difference is more evident in rural than in urban areas. Moreover, people who are poor are most likely to report financial cost as the reason for foregoing care when they have an illness, and this effect has increased with time. According to World Bank data (2014) OOP expenditure in Health is 62.42% of total health expenditure. Overall health expenditure is 4.7% of GDP which constitutes 3.3% for private sector and 1.42% for public sector. Such a predominant OOP expenditure in health is indicative of a highly regressive health care system as well as poverty.1 Studies have identified that too much reliance on OOP health payments at the time of care, in health care financing context dominated by private expenditures combined with weak public health systems, and almost negligible health insurance are largely responsible for high prevalence of catastrophic health payments in South Asia.5,6,7 There is a growing body of evidence that payments for health care thus can easily become a catastrophic health expenditure, especially when the public health care system is weak or unattractive, and poor people have to make use of private services.8,9
The present PAPer aims to estimate the treatment effect of Health insurance plans on the incidence of OOP health expenditure in India.
Materials and Methods:
Study design : Cross-Sectional study.
Data source: The study used 71st round of National Sample Survey (NSS) data from January to June 2014 on social consumption section 25.0. 71st round of NSSO covered the whole of the Indian Union. It used a multi stage sampling with 65932 households and 333104 persons. The main dependent variable is out-of-pocket expenditure (Y).
Out-of-pocket expenditure (OOP): This variable measures the value of out-of-pocket expenditure in health incurred by the person. It is calculated as the difference between total medical expenditure and the amount reimbursed for a person. Total medical expenditure consists of doctors’ fees, medicine cost, cost of diagnostic tests, other medical expenses, transport cost for the patients and other cost for treatment. In calculation logarithm of this variable (OOP) has been taken as the values of the variable were highly skewed.
Univariate and bivariate analysis has been used to see the distribution of health insurance coverage in India with respect to selected background characteristics. To investigate the variation in coverage at different level of sampling, multilevel logistic regression analysis was performed and the intra class correlation coefficient was calculated to detect the variation. To estimate the treatment effect of health insurance plans on the occurrence of out-of pocket health expenditure at national level, ordinary least square method (OLS), and Instrumental Variable (IV) model and propensity score matching (PSM) were employed in the study.
OLS MODEL-First of all I applied linear model with a basic econometric specification to analyze the effect of insurances on the occurrence of OOP health expenditure.
Y=X1b1+X2b2+......….+Xnbn+TjCj +ei….......eq(1)
Where Y is the Out-of-pocket expenditure, Xi are the covariates and T is the enrolment into health insurance. In this first model I assumed exogeneity, ie. The covariates are not correlated with the error term and thus I could directly estimate eq(1) independently.
IV MODEL: Considering endogenous treatment variables, I also used instrumental variable model with the traditional two-stage least square (2SLS) approach. The general approach in instrumental variable model with binary endogenous variable is to consider a latent variable model. Let y1 depend in part on y2, a binary endogenous regressor. We introduce an unobserved latent variable, y2* that determines whether y2=1 or 0. Then the model will be
Y1=b1y2+ b2X1+u
Y2*=X1c1+ X2c2 + v
Y2= 1 if Y2*>0, and 0 otherwise
The errors u and v are assumed to be correlated bivariate normal with Var(u)= σ, Var (v)=1 and Cov (u, v) = ρ*σ2. If covariance between the error terms comes to be 0, we can say that the errors are independent and thus no endogeneity exists. STATA 13 by default performs the endogeneity test while running the first stage regression. To find the appropriate covariates, I have searched the literature and found the main determinants of OOP health expenditure are economic status, sex, religion, social caste, and educational level, place of residence, chronic ailments, health insurance coverage, and marital status. As per econometric theory I used estimated value of the endogenous variable as the instrumental variable which is the best instrumental variable.
PSM MODEL: Using this model I estimated the average treatment effect (ATE) in the case of continuous outcome variable OOP. ATE can be defined as the mean difference in outcome among treated and control group. For the calculation of average treatment effect a counterfactual model has been constructed. Counterfactual is the potential outcome, or the state of affairs that would have happened in the absence of the cause. With the help of the counterfactual model, Average Treatment Effect on the Treated (ATT) has been calculated. This measure the impact of the treatment on treated
ATT= E [Y|D = 1] = E [Y1|D = 1] – E [Y0|D = 1]
Where E [Y1|D = 1] is the average outcome of the treated group. E [Y0|D = 1] is the counterfactual, it shows average outcome that the treated individuals would have obtained in the absence of treatment, which is unobserved. After that with the help of boot strapping method, using 100 replications the standard error of difference of average treatment effects was calculated.
Similarly we can calculate Average treatment effect on Untreated (ATU) which is also a counterfactual model. ATU can be defined as the impact of the treatment on the untreated, i.e. It measures what would be the effect of the treatment on the untreated group if they were treated.
ATU = E [Y|D = 0] = E [Y1|D = 0] – E [Y0|D = 0]
Results
It has been seen that as high as 86% of rural population and 82% of urban population were still not covered under any scheme of health expenditure support. It is also observed that such coverage was broadly correlated with levels of living in both rural and urban sector. The relationship seems to be stronger in the urban areas than in the rural areas. The values reflect a steady increase in the proportion of coverage by some scheme of health expenditure support with a rise in UMPCE level. On the whole, the poorer households appear not to recognize the efficacy of the coverage, both in rural and urban areas. Government, however, was able to bring about 12% urban and 13% rural populations under health protection coverage through Rastriya Swastika BimaYojana (RSBY) or similar plans. Only 12% households of 5th quintile class of urban area had some arrangement of medical insurance from private provider. For all others the share is negligible. (NSSO 2014). Table-1 shows the percentage distribution of health insurance coverage by selected characteristics of the respondents. From the percentage distribution of health insurance coverage we can say that male is more covered by any schemes of health insurance than female. But the difference is very small. This is also supported by the proportional distribution with respect to the sex. Almost equal proportion of male and female have health insurance. The corresponding confidence intervals are non-overlapping and the standard errors are very less. Corresponding Chi square value suggests that association is significant at 10% level of significance. With respect to the different social groups, the percentages of enrolment vary over the groups. Schedule tribes are more enrolled than other groups. Even proportionally STs is covered by a greater degree than others. Here also the standard errors are very less for all groups. Here also the association is significant at 5% level of significance. 34.1% of covered people belong to the Christian community which is much higher than other communities followed by Hindus (17.9%) and Islam (11.3%). Almost 29.7% Christians are covered by health insurance while the incidence is 15.8% and 10.5% among Hindus and Islam respectively. It can be said that education and health insurance coverage are positively related. Incidence of enrolment is much higher among highly educated people than people with lower academic degrees. Percentage distribution of the health insurance coverage indicates that most of the insured people are with lower academic degree. In India the mean family size is three. Household size has an important role on health insurance coverage. People from smaller family size (generally less than 6) are more likely to have health insurance than people from bigger families. Health insurance coverage differs with the place of residence. Urban people are more prone to enrolment than rural people. Even 18% urban people are under coverage while the same is only 14% for rural people. Health insurance coverage changes with the economic gradient. People belongs to highest quartile are more likely to be under any schemes than poor people. The result is satisfied by both the percentage and proportional distribution of health insurance coverage. Study suggests that never married people are more insured than currently married or widowed persons. But most of the insured people are currently married. From Table-2 we can say that 4% of the residual variance in the coverage of health insurance is explained due to the change in state while 5% is explained by the change in FSU (village). This may be the direct consequenceof high level of penetration of information among neighbouring villages.
Applying OLS, it is found that an insured person has to pay less than an uninsured person as OOP health expenditure. IV method also shows that an insured person has to pay less than an uninsured person as OOP health expenditure as presented in Table-3. The statistical software package STATA13 performs the endogeneity test by default when using “treatreg” command. If the error correlation ρ=0 then the errors are independent and there is no endogeneity problem. Here the LR test rejects the null hypothesis that ρ=0, thus health insurance is an endogenous variable. The correlation between the endogenous and instrumental variable is 0.2 which is low but not to flag up the problem of weak instrument. Even the F-statistics for the instrumental variable is 24.64 which is considerably larger than the rule of thumb value of 10. So the predicted value does not seem to be a weak instrument. PSM method estimates (Table-4) ATE -0.23 which means health insurance reduced the OOP health expenditure by an average of 1.26 Rs. Per head. From the estimated mean out-of-pocket health expenditure by different models (Table-5), we can see that in OLS model the mean OOP health expenditure is 5058.57 Rs for insured person when the same is 6063.55 Rs for an un-insured person. It shows that health insurance caused 2063.14 Rs less OOP health expenditure for an insured person on an average. The result varies significantly while IV model has been applied. It shows a reduction of 5136.97 Rs in OOP expenditure on an average for an insured person compared to an uninsured person. We can say that OLS suppress the effect of health insurance on the incidence of OOP health expenditure.
Discussion
It is found that an insured person has to pay less than an uninsured person as OOP health expenditure. The LR test rejects the null hypothesis that ρ=0, thus health insurance is an endogenous variable. PSM method estimates (Table-4) ATE -0.23 which means health insurance reduced the OOP health expenditure by an average of 1.26 Rs. Per head. From the estimated mean out-of-pocket health expenditure by different models (Table-5), we can see that in OLS model the mean OOP health expenditure is 5058.57 Rs for insured person when the same is 6063.55 Rs for an un-insured person. It shows that health insurance caused 2063.14 Rs less OOP health expenditure for an insured person on an average. The result varies significantly while IV model has been applied. It shows a reduction of 5136.97 Rs in OOP expenditure on an average for an insured person compared to an uninsured person. We can say that OLS suppress the effect of health insurance on the incidence of OOP health expenditure.
Limitation
This PAPer has some limitations. Health expenditure is, by definition, provisional to the utilization of health facilities. Thus, there is another possible econometric specification to take into account the endogeneity generated by health seeking behaviour. Such correction would require health status, clinical and outcomes data on nonusers to exogenously assess needed medical expenditures. Moreover, the present analysis does not include others indicators that can also describe the effect of the incidence of health expenditures on the household’s financial status, such as impoverishing expenditures. Moreover the effect of OOP health expenditure should be measured with respect to the ability to pay of the individual. Empirics of catastrophic healthcare expenditure, especially in the Indian context, are often based on consumption expenditure data that inadequately informs about the ability to pay. Use of such data can generate a pro-rich bias in the estimation of catastrophic expenditure thereby suggesting greater concentration of such expenditures among richer households.10-12
Supporting File
References
1. William Joe, Distressed financing of household out-of-pocket health care payments in India: incidence and correlates Health Policy and Planning 2015;30:728–741.
2. Jayakrishnan T, JeejaM ,Kuniyil V and Paramasivam S. 2016. Increasing Out-OfPocket Health Care Expenditure in India-Due to Supply or Demand?.100-105
3. Berki S. 1986. A Look at Catastrophic Medical Expenses and the Poor. Health Affairs. 5(4). pp. 138- 145.
4. Russell S. 1996. Ability to Pay for Health Care: Concepts and Evidence. Health Policy and Planning. 11 (3). pp 219-237.
5. Van Damme W, van Leemput L, Por I, Hardeman W, Meessen B. 2004. Outof-pocket health expenditure and debt in poor households: evidence from Cambodia. Tropical Medicine and International Health 9: 273–80
6. Wagstaff A, van Doorslaer E. 2003. Catastrophe and impoverishment in paying for health care: with applications to Vietnam, 1993–98. Health Economics 12: 921–34.
7. Owen O’Donnell, Eddy van Doorslaer, Adam Wagstaff, Magnus Lindelow. Analyzing health equity using household survey data : a guide to techniques and their implementation. WBI Learning Resources Series. The World Bank Washington, D.C. chapter 18 &19.
8. Pradhan M & Prescott N (2002) Social risk management options for medical care in Indonesia. Health Economics 11, 431–446
9. Kawabata K, Xu K &Carrin G (2002) Preventing impoverishment through protection against catastrophic health expenditure. Bulletin of the World Health Organization 80, 612.
10. Gupta I, Joe W. 2013. Refining estimates of catastrophic healthcare expenditure: an application in the Indian context. International Journal of Health Care Finance and Economics 13: 157–72
11. A.K. Nandakumar, MukeshChawla,Maryam Khan.(January 2000). Utilization of Outpatient Care in Egypt and its Implications for the Role of Government in Health Care Provision, Vol28, issue1, , pages 187-196.
12. Berman P, Ahuja R, Bhandari L. 2010. The impoverishing effect of healthcare payments in India: new methodology and findings. Economic and Political Weekly Vol. XLV: 65–71.